Modern BI tools promise a lot — faster insights, cleaner visuals, empowered users.
But here’s the truth from the trenches:
BI adoption fails when tools come before trust.
We’ve helped organizations untangle BI rollouts that looked good on paper but missed the mark in practice. And time after time, it wasn’t the tool’s fault. It was the approach.
When BI Modernization Stalls
At one State Fund, Tableau had been chosen as the flagship BI tool. On the surface, it made sense: sleek dashboards, interactive visuals, and wide industry adoption.
But within weeks, adoption began to slow. Reports that had been workhorses for years were suddenly out of reach — or worse, rebuilt in ways that didn’t serve the business.
Two examples stood out:
- Contingent Commission: A regulatory report with complex conditional formatting, pixel-perfect layout, and business rule-based rendering. Tableau struggled to deliver both the structure and export fidelity required for compliance.
- Agency Current Book: A report built with page sets, custom summary rows, and advanced crosstab logic — again, poorly suited to Tableau’s flat design model.
And then there was the OCR (Open Claims Review) — a report designed for live meetings with outside agents.
For OCR, the business needed to override data values temporarily, reflecting last-minute changes that wouldn’t hit the source system for up to an hour. This meant:
- Users could write back changes in the source system.
- The DW received a near-real-time push of just this data item.
- Cognos picked it up immediately, ensuring the report reflected real-time adjustments.
Tableau, by contrast, required a full refresh to pick up those changes — not viable for these time-sensitive meetings.
From Feature List to Data Wishlist
We shifted the conversation from features to needs.
We led the team through a data wishlist process:
- What absolutely needed to stay?
- What could evolve?
- What was worth building new?
With that in hand, we ran a gap analysis and helped architect a hybrid BI environment:
- Cognos handled high-complexity reports, real-time overrides, and print-perfect outputs.
- Tableau supported exploratory dashboards and visual summaries where its strengths shone.
It wasn’t about holding onto the past. It was about preserving trust — and designing BI around how people worked, not just how the tool was sold.
BI Migration Pitfalls We See Again and Again:
- Tool-first thinking: Choosing a platform before understanding user needs.
- Feature-for-feature rebuilds: Replicating old reports exactly, even if they weren’t ideal to begin with.
- Ignoring data flow realities: Real-time needs vs. batch refresh capabilities.
- One-size-fits-all UX: Assuming executives and analysts want the same thing.
Successful BI isn’t about adopting what’s next — it’s about elevating what works and building for what’s possible.
Lessons in UX from the Field
Across industries, we’ve seen the same patterns:
- At a regional public university system, HR dashboards were redesigned around what leaders did with the data — not just how they wanted it to look.
- At a global retail group, dashboards were tailored for regional exploration and executive summaries, respecting different users’ needs.
- At a behavioral health provider, manual Excel processes were automated and brought back into the BI platform, improving time to insight.
Our UX Framework for BI Adoption
We’ve distilled years of experience into a simple model for BI success:
Datagize UX Triangle
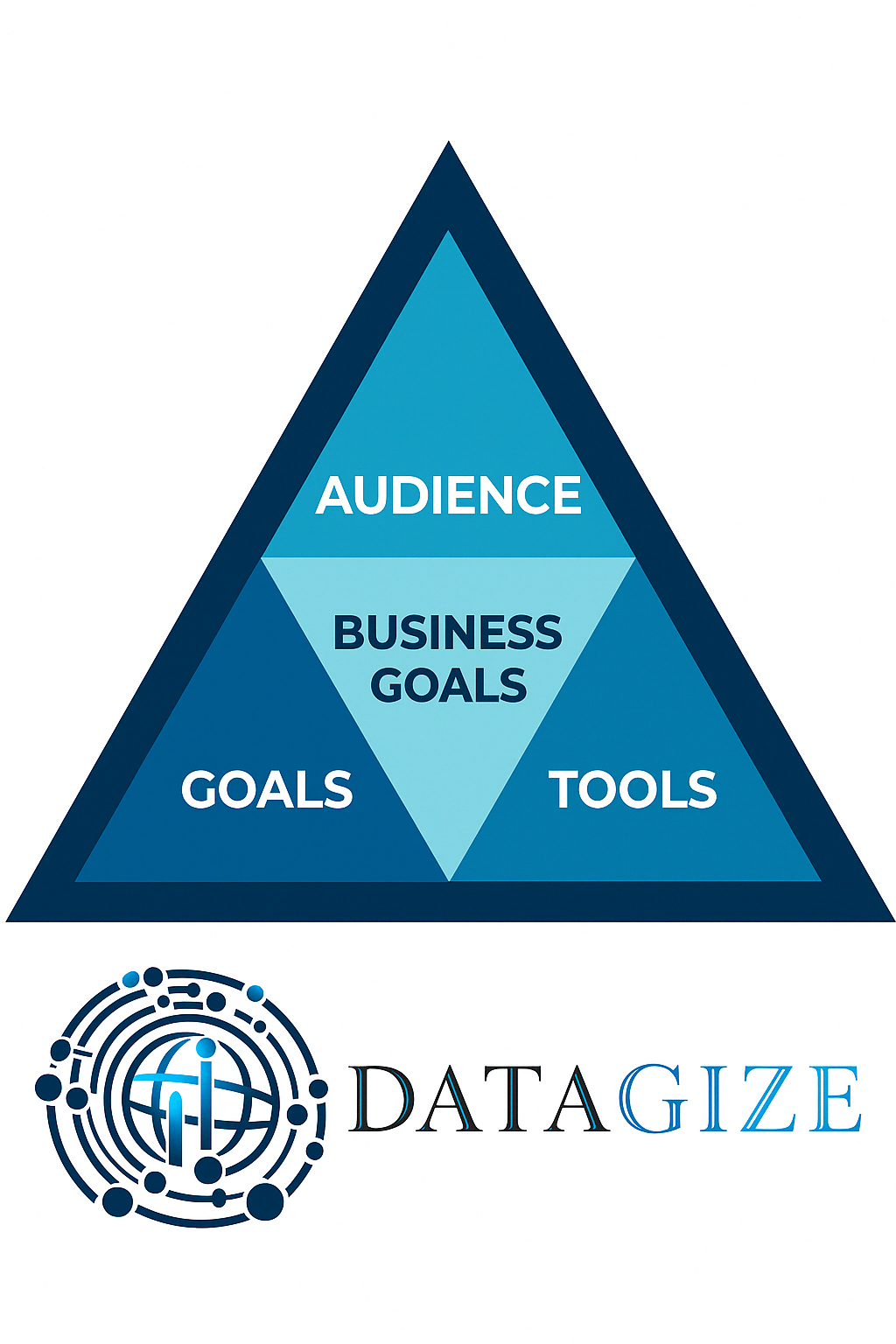
- Audience: Who is using the data? What do they need to know quickly?
- Purpose: What action does this report drive? Storytelling or exploration?
- Tool: Which platform supports this best — structurally and visually?
UX Drives Trust. Trust Drives Adoption.
The best BI projects don’t start with tools — they start with users.
If your dashboards aren’t landing, or your adoption is stalling, it’s time to step back. Rethink who you’re designing for, not just what you’re building.
A Personal Note from the Trenches
When I first started building BI solutions in the ‘90s, it was all about getting the reports out — making sure leadership had what they asked for.
But I learned quickly: unless the people on the ground trust the data and know how to use it, none of it matters.
Over the years, I’ve built systems for sales, supply chain, finance, marketing, manufacturing, claims, and more. The tools have changed — but the challenge hasn’t.
BI adoption isn’t a tech problem. It’s a UX problem.
And that’s where we come in.
Ready to Rethink Your BI UX?
If you’re about to choose a tool, or already mid-migration, take a step back.
We’re offering a limited number of free 60-minute BI Trust & UX Fit consults this month.
Let’s talk about how UX could save you months — and rebuild trust before it’s lost.
You bring the road.
We’ll bring the steering wheel.